The Future of AI in Radiography : Opportunities and Challenges
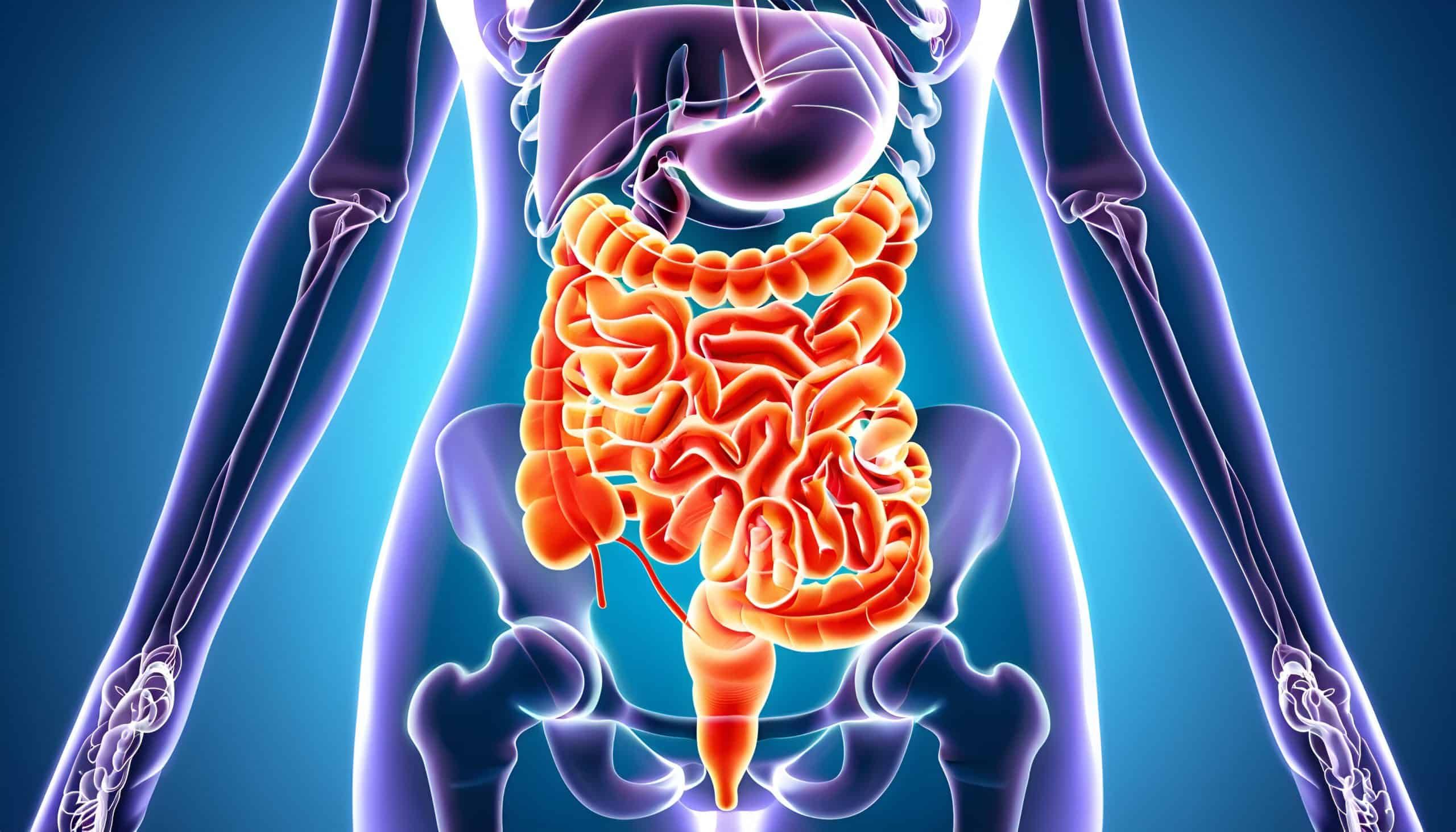
Artificial intelligence (AI) is swiftly transforming various industries, and radiography is no exception. As we advance further into the 21st century, the fusion of AI technologies with radiographic practices promises to revolutionize how radiologists interpret images, diagnose conditions, and interact with patients. This article delves into both the thrilling opportunities and significant challenges that AI presents in the field of radiology, particularly focusing on X-ray and mammography technologies.
[ez-toc]
Revolutionizing Diagnostic Accuracy
The integration of Artificial Intelligence (AI) within the field of radiology, especially noted in specialized areas like X-ray and mammography, is setting new standards for diagnostic precision. AI algorithms are not merely tools but transformational technologies that analyze extensive imaging data with remarkable accuracy. These algorithms have the unique capability to detect complex patterns and anomalies in imaging data—subtleties that may often be overlooked by the human eye.
This technological advancement means that radiographers and X-ray techs are witnessing a significant decline in diagnostic errors. More importantly, AI facilitates a much quicker diagnostic process. The speed and accuracy provided by AI do not just improve operational efficiency but critically enhance patient care. Faster and more precise diagnoses lead to quicker treatment decisions, which can be life-saving in acute medical scenarios.
Moreover, AI’s deep learning capabilities allow for the continuous improvement of diagnostic tools. As these systems are fed more data, their ability to interpret and understand complex medical images evolves, leading to ever-improving diagnostic support. In fields like oncology, early and accurate detection of tumors through enhanced imaging techniques can significantly impact patient outcomes, highlighting the crucial role of AI in modern medicine.
Additionally, the application of AI extends into predictive analytics, which plays a vital role in forecasting disease progression and potential health crises before they become evident clinically. This predictive power of AI is crucial for proactive patient management, particularly in managing chronic conditions and preemptive medical interventions. For more insights into how AI is shaping the future of radiology, explore industry perspectives on the transformative capabilities of AI in healthcare.
1. Enhanced Image Interpretation
Machine learning models within AI frameworks have revolutionized how radiographic images are interpreted. By training on vast datasets comprising thousands of annotated images, these models continuously improve their diagnostic precision. This capability is particularly transformative in radiography, where the early detection of conditions such as cancers, fractures, and other abnormalities can significantly influence treatment outcomes.
The application of AI in image interpretation extends beyond mere identification; it involves a comprehensive analysis that characterizes each detected anomaly according to shape, size, and suspected pathology. This level of detail assists radiologists in assessing the severity and progression of diseases. For instance, in mammography, AI can detect microcalcifications and subtle masses that might not be as apparent in traditional analyses, thereby aiding in the early stages of breast cancer detection.
Furthermore, these AI-driven systems are equipped with learning algorithms that adapt to new findings and evolving medical knowledge, ensuring that their diagnostic capabilities improve over time. This adaptive learning process not only boosts the accuracy of diagnoses but also reduces the rate of false positives and negatives, streamlining the workflow for radiographers and enhancing patient care.
2. Reduced Radiation Exposure
AI can also optimize the process of taking radiographs, potentially reducing the radiation dose required during imaging. This is a critical consideration in radiography, where patient safety depends on minimizing exposure to radiation.
Streamlining Workflow in Radiology Departments
The integration of AI technologies in radiology is transforming more than just diagnostic accuracy; it is also revolutionizing the efficiency of entire radiology departments. By automating routine tasks, such as image sorting and preliminary analyses of standard cases, AI allows clinicians to allocate more time and resources to complex cases and direct patient care. This shift not only enhances the quality of care but also boosts departmental efficiency, creating a more streamlined health service delivery.
1. Automating Routine Tasks
AI excels in managing repetitive tasks that traditionally consume considerable time and effort in radiology departments. Functions such as sorting through thousands of diagnostic images, categorizing them, and performing initial diagnostic reviews are now increasingly handled by sophisticated AI systems. This automation significantly speeds up the diagnostic process, thereby reducing patient wait times and increasing the throughput of radiological examinations. Explore further details on how AI is enhancing radiographic practices.
2. Integration with Electronic Health Records (EHR)
AI’s capability extends beyond imaging and diagnostics into the realm of data integration. AI systems are increasingly being integrated with Electronic Health Records (EHR), enhancing the connectivity between different health information systems. This integration provides radiologists and other healthcare providers with a comprehensive view of a patient’s medical history, crucial for informed decision-making and crafting personalized care plans. Such comprehensive data integration leads to improved patient outcomes and optimized treatment pathways. For a deeper understanding of AI’s role in healthcare data management, see HealthIT’s overview of EHR systems.
Challenges Facing AI in Radiology
Despite the promising advancements, the integration of AI in radiology is not without its challenges.
1. Ethical and Legal Concerns
The rapid adoption of AI in radiology brings with it a host of ethical and legal challenges that need urgent addressing. Concerns around data privacy are paramount, as the use of AI involves handling large volumes of sensitive patient information. Ensuring that this data is protected and that the privacy of patients is respected is crucial, and radiologists must navigate complex regulations pertaining to data security. Explore more about the importance of ethical practices in radiography on our site.
Informed consent is another critical area impacted by AI. Patients must be adequately informed about how their data will be used in AI-driven diagnostics, what it means for their treatment, and how it might affect their privacy rights. Furthermore, the potential for bias in AI algorithms remains a significant concern. Bias can occur due to the datasets on which AI models are trained, often reflecting historical biases that can lead to unequal treatment outcomes among different demographic groups. Addressing these biases is essential for the ethical deployment of AI in healthcare.
Legal frameworks are still catching up with the advancements in AI technology, creating a grey area that complicates the widespread implementation of AI solutions in clinical settings. The evolving nature of these legal standards demands that healthcare providers remain constantly informed and compliant with the latest regulations. For further insights into current regulatory standards in healthcare AI, refer to the WHO’s guidelines on AI in health.
2. Resistance to Change
Change can be daunting, and the field of radiology is currently at a critical juncture with the integration of AI technologies. While the benefits of AI in enhancing diagnostic accuracy and streamlining workflows are clear, there is often substantial resistance among healthcare professionals. The shift from well-established traditional methods to AI-powered techniques can seem overwhelming, requiring not only new skills but also a new mindset.
Significant training and adjustment are necessary to facilitate this transition. Radiologists and technicians must become proficient with new software and algorithms, which demands ongoing education and practice. This can be particularly challenging in busy clinical environments where time is a premium. For resources on adapting to technological advances in radiology, visit our Continuing Education for Radiographers page.
Beyond the practical aspects, there’s also a psychological component to this resistance. Many practitioners may feel threatened by AI, fearing it might replace their roles or diminish their years of training and experience. However, it’s important to emphasize that AI is intended to complement, not replace, the critical thinking skills of radiologists. Addressing these fears through open dialogue, comprehensive training programs, and clear communication about AI’s role as an aid rather than a replacement is vital. For more on managing change in healthcare settings, consider exploring Health Affairs’ insights on change management.
3. High Initial Costs
The integration of AI into radiology is not just a technological upgrade but also a significant financial undertaking. Implementing AI technologies within radiology practices demands a robust initial investment that spans several areas. Firstly, the cost of software development or acquisition can be substantial. AI systems, especially those designed for diagnostic imaging, require sophisticated, high-performing algorithms that are often expensive to develop or purchase.
In addition to software costs, substantial investments are needed for hardware upgrades. Existing systems may need to be replaced or augmented to handle the intensive computational demands of AI applications. This can include faster processors, more robust servers, and specialized imaging hardware that are compatible with AI technology. For an overview of necessary hardware for radiology AI systems, visit our X-ray Tech Equipment page.
Finally, there is a significant cost associated with training personnel to effectively use these new AI systems. Ensuring that radiologists, technicians, and support staff are fully competent in utilizing AI requires not just initial training but ongoing education to keep up with continuous advancements in AI technology. For training resources, check out our Radiography CE courses.
The financial burden of these initial costs can be a barrier for many radiology departments, particularly those operating within smaller or resource-limited settings. However, the long-term benefits of AI, such as increased diagnostic accuracy, efficiency, and reduced long-term operating costs, often justify the initial investment. For a detailed analysis on the ROI of AI in healthcare, explore McKinsey’s report on healthcare AI investments.
The Road Ahead
As we peer into the future of radiology, the role of Artificial Intelligence (AI) is poised to expand even further, reshaping this critical medical field in profound ways. The potential benefits of AI, such as markedly improved diagnostic accuracy, elevated patient safety standards, and streamlined workflows, present compelling reasons for its continued adoption and development. These advantages promise not only to enhance the quality of healthcare delivery but also to transform the patient experience by reducing diagnostic times and increasing the accuracy of treatment plans.
However, the journey ahead is not without its challenges. Integrating AI into daily radiological practice requires a synchronized effort from multiple fronts: technology developers need to continue refining AI tools to ensure they are both effective and ethical, radiologists must embrace these advancements and adapt to new workflows, and regulatory bodies have to evolve policies that keep pace with technological developments. To explore how these roles intersect and enhance the adoption of AI in radiology, consider visiting our Mammography CE page for continuous education on the latest technology updates.
Furthermore, ongoing dialogue among all stakeholders in the healthcare ecosystem is essential. This includes engaging with patient advocacy groups to ensure that patient interests are always at the forefront of technological innovations. Collaboration across disciplines will be key to addressing the ethical, legal, and practical challenges that accompany the integration of AI into radiology. For broader perspectives on future technologies in healthcare, check out HealthIT’s vision on the future of care.
Conclusion
The journey of integrating AI into radiology is full of opportunities and challenges. By embracing AI, the field of radiography can look forward to enhanced diagnostic tools, improved patient outcomes, and more efficient processes. However, navigating the complexities of AI implementation will be crucial to fully realizing these benefits.
FAQs
- What are the main benefits of AI in radiography?AI enhances diagnostic accuracy, reduces patient exposure to radiation, and streamlines workflow by automating routine tasks.
- How does AI improve diagnostic accuracy in radiography?AI algorithms analyze imaging data to identify subtle patterns and anomalies, helping in early disease detection and reducing diagnostic errors.
- What are the ethical concerns associated with AI in radiography?Key concerns include patient data privacy, informed consent, and the potential for algorithmic bias, which must be carefully managed.
- Why is there resistance to adopting AI in radiography?Resistance often stems from the need for significant changes in practice, including training and adjustments in workflow, which can be daunting.
- What investments are needed to integrate AI into radiology departments?Investments include costs for AI software, hardware upgrades, and training for radiology staff to adapt to new technologies.
- Can AI replace radiologists?No, AI is intended to augment the capabilities of X-Ray Techs, not replace them, by assisting with diagnosis and workflow efficiency.
Your thoughts on the future of AI in radiology are invaluable. What opportunities or challenges do you foresee? Share your views in the comments below or on social media, linking back to this article!
FAQs: The Future of AI in Radiography
- Q: How is AI currently being used in radiography?
- A: AI is currently being used in radiography for image analysis, assisting in the detection of abnormalities, automating repetitive tasks, and improving the accuracy of diagnoses. AI algorithms can quickly analyze imaging data to identify patterns that might be missed by the human eye.
- Q: What are the main benefits of integrating AI in radiography?
- A: The main benefits of integrating AI in radiography include increased efficiency in image analysis, reduction in human error, enhanced diagnostic accuracy, faster turnaround times for results, and the ability to handle large volumes of data. AI also assists radiologists by highlighting potential areas of concern, thus aiding in early diagnosis.
- Q: What challenges are associated with the implementation of AI in radiography?
- A: Challenges include high initial costs, the need for significant amounts of high-quality data to train AI systems, potential resistance from medical professionals, concerns over job displacement, and ensuring the ethical use of AI. Additionally, there’s a need for regulatory frameworks to ensure patient safety and data security.
- Q: How can radiographers prepare for the integration of AI in their field?
- A: Radiographers can prepare by staying informed about advancements in AI technology, engaging in continuous education and training, developing skills in data analysis and machine learning, and being open to collaborating with AI systems. It’s also important to understand the ethical considerations and the role of AI in enhancing patient care.
- Q: Will AI replace radiographers in the future?
- A: While AI will significantly transform radiography, it is unlikely to replace radiographers entirely. Instead, AI will augment the capabilities of radiographers, allowing them to focus on more complex tasks that require human judgment and expertise. The human touch and clinical experience will remain crucial in patient care.
- Q: What ethical considerations must be taken into account with AI in radiography?
- A: Ethical considerations include ensuring patient privacy and data security, preventing biases in AI algorithms, obtaining informed consent for AI-assisted procedures, and maintaining transparency in AI decision-making processes. It is also important to address issues related to accountability and the impact of AI on employment in the radiography field.